Secure ML Library
Secure ML Research Tutorial: Wild Patterns Secure ML Library

SecML has been partially developed with the support of European Union’s ALOHA project
Horizon 2020 Research and Innovation programme, grant agreement No. 780788.
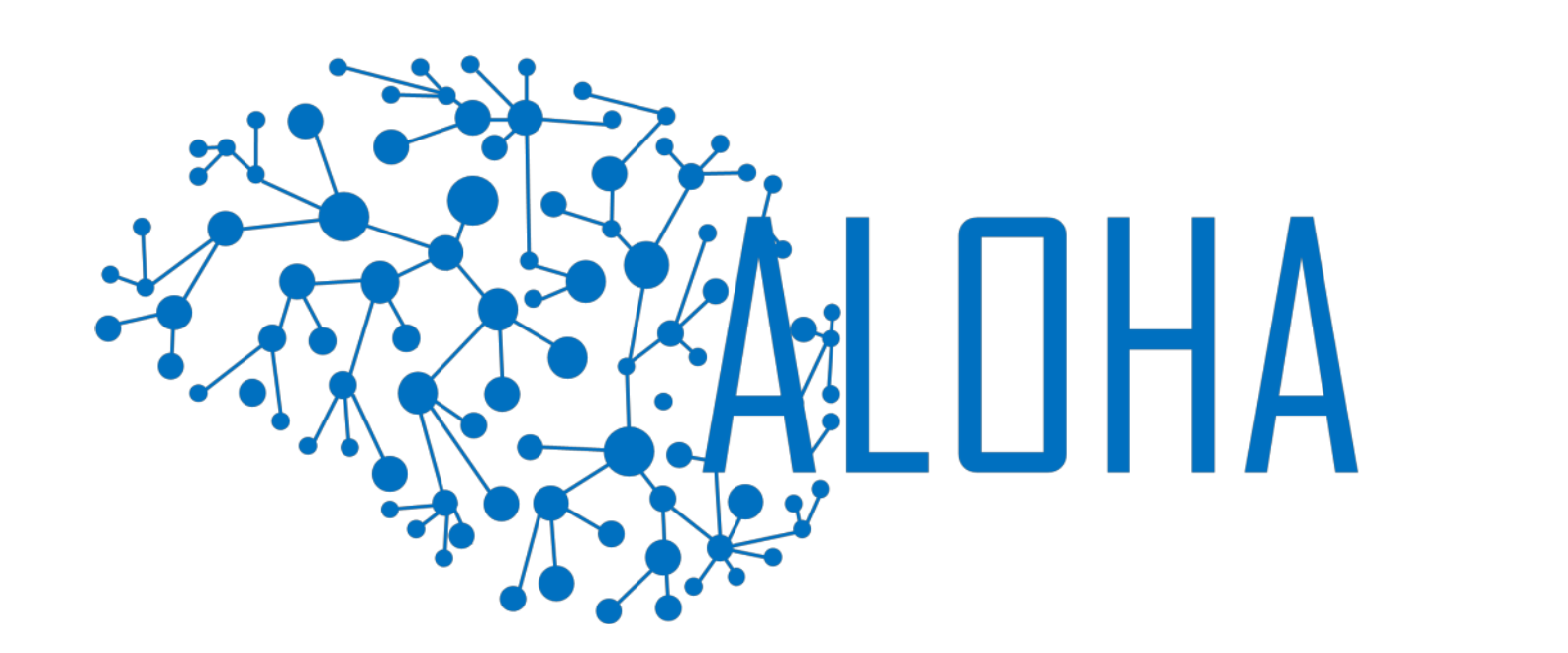
It comes with a set of powerful features:
-
Dense/Sparse data support. We provide full, transparent support for both dense (through
numpy
library) and sparse data (throughscipy
library) in a single data structure. -
Wide range of supported ML algorithms. All supervised learning algorithms supported by
scikit-learn
are available, as well as Neural Networks (NNs) through PyTorch deep learning platform (coming soon). -
Built-in attack algorithms. Evasion and poisoning attacks based on a custom-developed fast solver.
-
Visualize your results. We provide visualization and plotting framework based on the widely-known library matplotlib.
-
Explain your results. Explainable ML methods to interpret model decisions via influential features and prototypes. (coming soon)
-
Extensible. Easily create new wrappers for ML models or attack algorithms extending our abstract interfaces.
-
Multi-processing. Do you want to save time further? We provide full compatibility with all the multi-processing features of
scikit-learn
andpytorch
, along with built-in support of the joblib library.

Authors
-
Marco Melis (maintainer) [1]
-
Ambra Demontis [1]
-
Maura Pintor [1], [2]
-
Battista Biggio [1], [2]
Credits
-
numpy
Travis E, Oliphant. “A guide to NumPy”, USA: Trelgol Publishing, 2006. -
scipy
Travis E. Oliphant. “Python for Scientific Computing”, Computing in Science & Engineering, 9, 10-20, 2007. -
scikit-learn
Pedregosa et al., “Scikit-learn: Machine Learning in Python”, JMLR 12, pp. 2825-2830, 2011.
Copyright
SecML has been developed by PRALab - Pattern Recognition and Applications lab and Pluribus One s.r.l. under Apache License 2.0. Copyright 2019.
If you encounter any bug, please report them using the GitLab issue tracker.
More info here.